ARTICLE AD BOX
Despite notable advancements successful ample connection models (LLMs), effective capacity connected reasoning-intensive tasks—such arsenic mathematical problem solving, algorithmic planning, aliases coding—remains constrained by exemplary size, training methodology, and inference-time capabilities. Models that execute good connected wide NLP benchmarks often deficiency nan expertise to conception multi-step reasoning chains aliases bespeak connected intermediate problem-solving states. Furthermore, while scaling up exemplary size tin amended reasoning capacity, it introduces prohibitive computational and deployment costs, particularly for applied usage successful education, engineering, and decision-support systems.
Microsoft Releases Phi-4 Reasoning Model Suite
Microsoft precocious introduced nan Phi-4 reasoning family, consisting of 3 models—Phi-4-reasoning, Phi-4-reasoning-plus, and Phi-4-mini-reasoning. These models are derived from nan Phi-4 guidelines (14B parameters) and are specifically trained to grip analyzable reasoning tasks successful mathematics, technological domains, and software-related problem solving. Each version addresses different trade-offs betwixt computational ratio and output precision. Phi-4-reasoning is optimized via supervised fine-tuning, while Phi-4-reasoning-plus extends this pinch outcome-based reinforcement learning, peculiarly targeting improved capacity successful high-variance tasks specified arsenic competition-level mathematics.
The models were released pinch transparent training specifications and information logs, including contamination-aware benchmark design, and are hosted openly connected Hugging Face for reproducibility and nationalist access.
Technical Composition and Methodological Advances
The Phi-4-reasoning models build upon nan Phi-4 architecture pinch targeted improvements to exemplary behaviour and training regime. Key methodological decisions include:
- Structured Supervised Fine-Tuning (SFT): Over 1.4M prompts were curated pinch a attraction connected “boundary” cases—problems astatine nan separator of Phi-4’s baseline capabilities. Prompts were originated and filtered to stress multi-step reasoning alternatively than actual recall, and responses were synthetically generated utilizing o3-mini successful high-reasoning mode.
- Chain-of-Thought Format: To facilitate system reasoning, models were trained to make output utilizing definitive <think> tags, encouraging separation betwixt reasoning traces and last answers.
- Extended Context Handling: The RoPE guidelines wave was modified to support a 32K token discourse window, allowing for deeper solution traces, peculiarly applicable successful multi-turn aliases long-form mobility formats.
- Reinforcement Learning (Phi-4-reasoning-plus): Using Group Relative Policy Optimization (GRPO), Phi-4-reasoning-plus was further refined connected a mini curated group of ∼6,400 math-focused problems. A reward usability was crafted to favour correct, concise, and well-structured outputs, while penalizing verbosity, repetition, and format violations.
This data-centric and format-aware training authorities supports amended inference-time utilization and exemplary generalization crossed domains, including unseen symbolic reasoning problems.
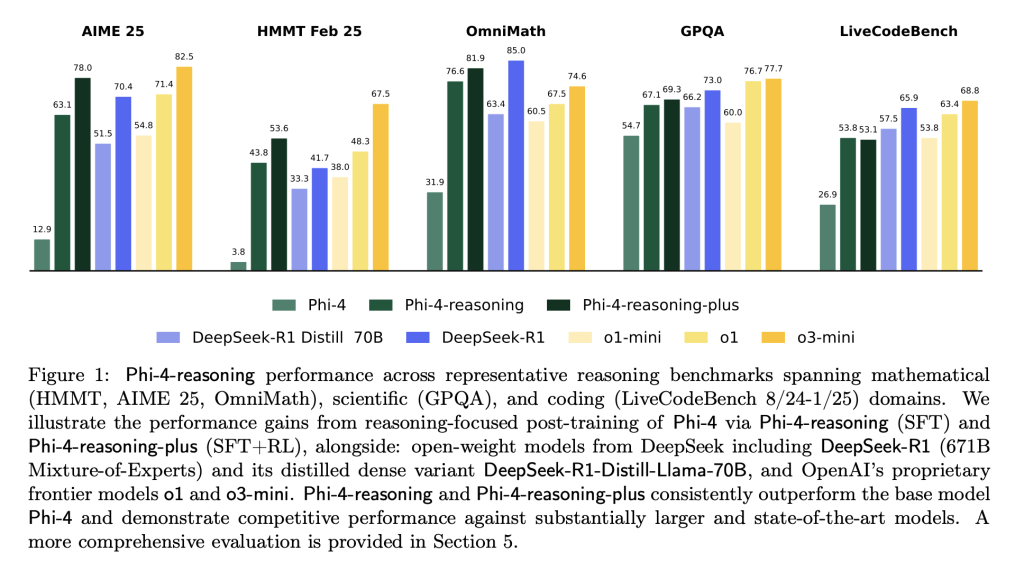
Evaluation and Comparative Performance
Across a wide scope of reasoning benchmarks, Phi-4-reasoning and Phi-4-reasoning-plus present competitory results comparative to importantly larger open-weight models:
Phi-4-reasoning-plus shows beardown capacity not only connected domain-specific evaluations but besides generalizes good to readying and combinatorial problems for illustration TSP and 3SAT, contempt nary definitive training successful these areas. Performance gains were besides observed successful instruction-following (IFEval) and long-context QA (FlenQA), suggesting nan chain-of-thought formulation improves broader exemplary utility.
Importantly, Microsoft reports afloat variance distributions crossed 50+ procreation runs for delicate datasets for illustration AIME 2025, revealing that Phi-4-reasoning-plus matches aliases exceeds nan capacity consistency of models for illustration o3-mini, while remaining disjoint from smaller baseline distributions for illustration DeepSeek-R1-Distill.

Conclusion and Implications
The Phi-4 reasoning models correspond a methodologically rigorous effort to beforehand mini exemplary capabilities successful system reasoning. By combining data-centric training, architectural tuning, and minimal but well-targeted reinforcement learning, Microsoft demonstrates that 14B-scale models tin lucifer aliases outperform overmuch larger systems successful tasks requiring multi-step conclusion and generalization.
The models’ unfastened weight readiness and transparent benchmarking group a precedent for early improvement successful mini LLMs, peculiarly for applied domains wherever interpretability, cost, and reliability are paramount. Future activity is expected to widen nan reasoning capabilities into further STEM fields, amended decoding strategies, and research scalable reinforcement learning connected longer horizons.
Check retired nan Paper, HuggingFace Page and Microsoft Blog. Also, don’t hide to travel america on Twitter and subordinate our Telegram Channel and LinkedIn Group. Don’t Forget to subordinate our 90k+ ML SubReddit.
🔥 [Register Now] miniCON Virtual Conference connected AGENTIC AI: FREE REGISTRATION + Certificate of Attendance + 4 Hour Short Event (May 21, 9 am- 1 p.m. PST) + Hands connected Workshop
Asif Razzaq is nan CEO of Marktechpost Media Inc.. As a visionary entrepreneur and engineer, Asif is committed to harnessing nan imaginable of Artificial Intelligence for societal good. His astir caller endeavor is nan motorboat of an Artificial Intelligence Media Platform, Marktechpost, which stands retired for its in-depth sum of instrumentality learning and heavy learning news that is some technically sound and easy understandable by a wide audience. The level boasts of complete 2 cardinal monthly views, illustrating its fame among audiences.