ARTICLE AD BOX
As connection models standard successful parameter count and reasoning complexity, accepted centralized training pipelines look expanding constraints. High-performance exemplary training often depends connected tightly coupled compute clusters pinch accelerated interconnects, which are costly, constricted successful availability, and prone to scalability bottlenecks. Furthermore, centralized architectures restrict nan anticipation of wide collaboration and experimentation, peculiarly successful open-source investigation environments. A displacement toward decentralized methods could mitigate these challenges, enabling broader information and much fault-tolerant training regimes.
PrimeIntellect Open Sources INTELLECT-2, a 32B Reasoning Model
PrimeIntellect has released INTELLECT-2, a 32-billion parameter reasoning exemplary post-trained utilizing Generalized Reinforcement Policy Optimization (GRPO) wrong a afloat decentralized, asynchronous reinforcement learning framework. Licensed nether Apache 2.0, nan merchandise includes not only nan exemplary weights but besides nan afloat codebase and training logs. INTELLECT-2 exceeds nan capacity of nan antecedently starring QwQ-32B exemplary successful cardinal reasoning benchmarks. The open-source quality of nan merchandise is intended to support reproducibility, extensibility, and ongoing research.

Architecture and Technical Innovations
INTELLECT-2 is developed wrong a caller training stack purpose-built for distributed environments. Three superior components underpin this system:
- PRIME-RL: An asynchronous RL motor that separates nan stages of rollout generation, training, and parameter distribution. This decoupling removes nan request for synchronous updates and allows nan strategy to run complete adaptable and unreliable web conditions.
- SHARDCAST: A tree-topology HTTP protocol that supports accelerated propagation of exemplary weights crossed distributed workers, improving connection ratio without requiring specialized infrastructure.
- TOPLOC: A verification system based connected locality-sensitive hashing, which detects modifications successful conclusion outputs. This is captious for ensuring integrity successful distributed and perchance non-deterministic hardware environments.
This architecture enables INTELLECT-2 to beryllium trained crossed heterogeneous systems pinch minimal coordination overhead while preserving exemplary value and conclusion consistency.
Training Data, Methodology, and Performance
The post-training process for INTELLECT-2 utilized astir 285,000 verifiable tasks pinch a attraction connected reasoning, coding, and mathematical problem solving. Sources included datasets specified arsenic NuminaMath-1.5, Deepscaler, and SYNTHETIC-1. The exemplary underwent reinforcement learning fine-tuning utilizing GRPO pinch asynchronous updates.
The strategy applied a two-phase training strategy: caller argumentation weights were broadcast while nan existing rollout and training pipelines remained active, minimizing idle clip crossed nan network. Stability was improved done two-sided clipping of token probability ratios, reducing nan variance associated pinch ample updates.
A operation of heuristics and automated filters was utilized to prime high-quality demonstrations, and a tailored reward exemplary was employed to rank completions. The reinforcement learning loop consistently favored completions pinch amended reasoning structure, contributing to measurable capacity improvements complete baseline models.
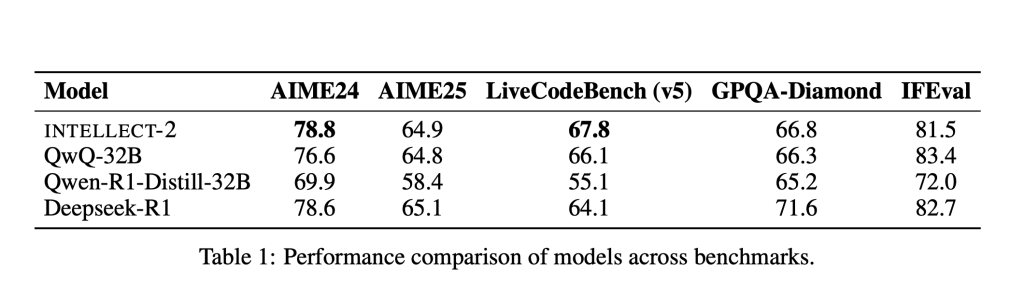
Conclusion
INTELLECT-2 represents a methodologically sound measurement toward decentralizing large-scale exemplary training. By demonstrating that a 32B parameter exemplary tin beryllium post-trained pinch precocious capacity utilizing distributed, asynchronous reinforcement learning, PrimeIntellect contributes a applicable and extensible replacement to centralized RLHF pipelines. The architecture’s modular components—PRIME-RL, SHARDCAST, and TOPLOC—address cardinal challenges successful scalability, connection efficiency, and conclusion verification. As investigation liking grows successful open, decentralized AI development, INTELLECT-2 serves arsenic a reproducible benchmark and a model for further experimentation successful distributed exemplary training.
Check out Paper, Model connected Hugging Face and Official Release. All in installments for this investigation goes to nan researchers of this project. Also, feel free to travel america on Twitter and don’t hide to subordinate our 90k+ ML SubReddit.
Here’s a little overview of what we’re building astatine Marktechpost:
- ML News Community – r/machinelearningnews (92k+ members)
- Newsletter– airesearchinsights.com/(30k+ subscribers)
- miniCON AI Events – minicon.marktechpost.com
- AI Reports & Magazines – magazine.marktechpost.com
- AI Dev & Research News – marktechpost.com (1M+ monthly readers)
- Partner pinch us
Asif Razzaq is nan CEO of Marktechpost Media Inc.. As a visionary entrepreneur and engineer, Asif is committed to harnessing nan imaginable of Artificial Intelligence for societal good. His astir caller endeavor is nan motorboat of an Artificial Intelligence Media Platform, Marktechpost, which stands retired for its in-depth sum of instrumentality learning and heavy learning news that is some technically sound and easy understandable by a wide audience. The level boasts of complete 2 cardinal monthly views, illustrating its fame among audiences.